Marketing mix modelling has been around as a technique or a tools for quite some time but as of my experience was always a niche topic for big global brands and as well a little too complicated for the average marketeer. There are a few providers like Kantar that offer solutions and also consulting in this area. But recently there has been more movement and development in this area as big digital platform players like Google and Meta also tap into this territory. With offering open source solution for MMM they are trying to close the gap of truly understanding marketing spending that web analytics and attribution approaches are not able to fill.
What is marketing mix modelling?
Let’s start from the very beginning: what is this MM? Marketing Mix Modeling (MMM), also known as econometrics, is a method that uses advanced statistical analysis on various variables – including advertising campaigns, pricing, weather conditions, and economic factors – to determine the correlation between these factors and increased sales and profits over a longer period. The goal is to understand how these variables can be used optimally.
Unlike approaches like Multi-Touch Attribution (MTA), which uses user-level tracking over shorter periods and primarily digital channels, MMM relies on aggregated data over weeks, months, or even years. It considers various types of marketing interventions
While both MMM and MTA have similar objectives, they are tactically distinct. MTA employs user-level tracking across a shorter period, primarily across digital channels, to calculate ROAS and optimize budget allocation accordingly. This has sometimes led to an over-reliance on last-click attribution, which is increasingly criticized. Her is an self explaining overview of typical measurement approaches in marketing:
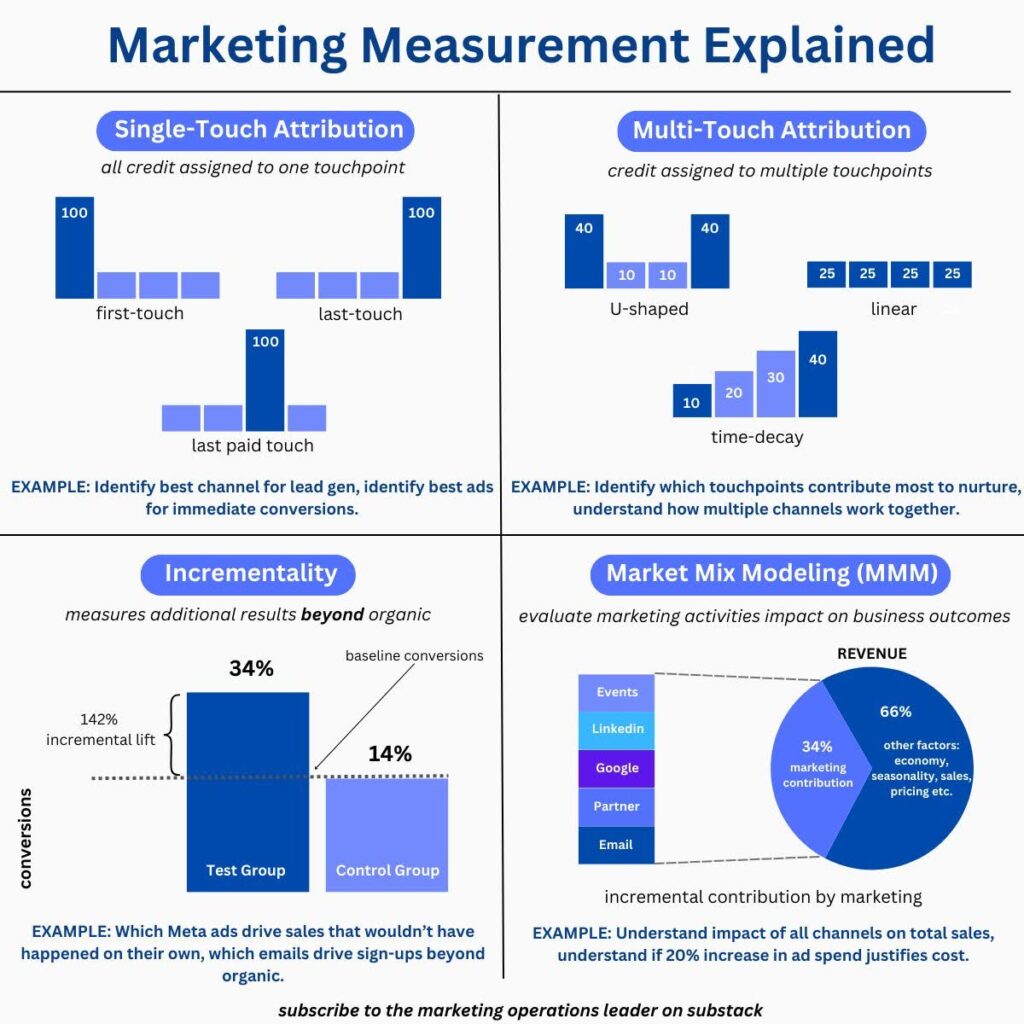
Recently attribution solution got under serious pressures: firstly the deprecation of the 3rd party cookies in browsers make this models that rely heavily on tracking cookies and other web tech very inaccurate as well as attribution modelling in general was accused to not represent a realistic picture and very much favour big digital platform, who have mostly pushed that solutions into the marketing world. Now with the criticism of attribution solutions the big digital platform companies try to offer their marketeers new solution to balance their budgets in the right way.
The engagement of the digital platforms
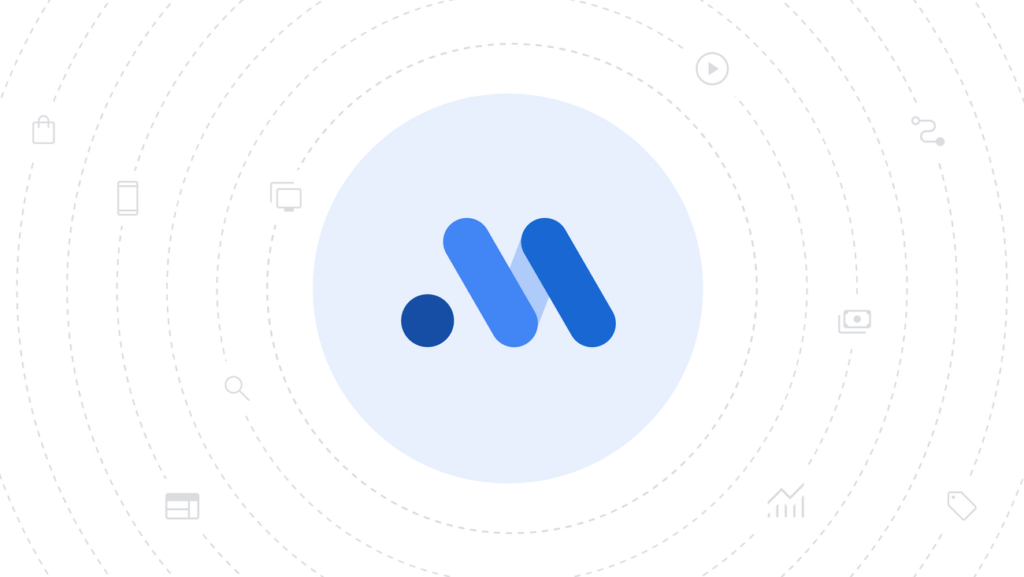
Companies like Google with its open-source platform Meridian and Meta with Robyn have recently introduced open-source MMM solutions to provide more transparency and allow data teams to customize and refine their models. Meridian, for example, aims to help marketers measure performance across various media by using Bayesian causal inference models. Google especially is investing remarkable effort in bringing their MMM into the market with offering a Partner Program and have very known marketing player and networks on board right away from the start. So we can see that they have legit interest that people start using their MMM solution. Critics might assume that Meridian somehow favours Google channels in their output in order to strengthen their market position, but as Meridian is an open source solution its internals are fully transparent viewable and can be investigated by the community.
The open source solution from Google and Meta can be a good starting point for brands and their agencies to tap into the field of MMM. Also with being open this is a big opportunity for smaller agencies or teams that want to specialize in this emerging area.
How to succeed with MMM?
Like most complex solution MMM requires profound skills in marketing but also knowledge in advanced statistics and a solid tool expertise.
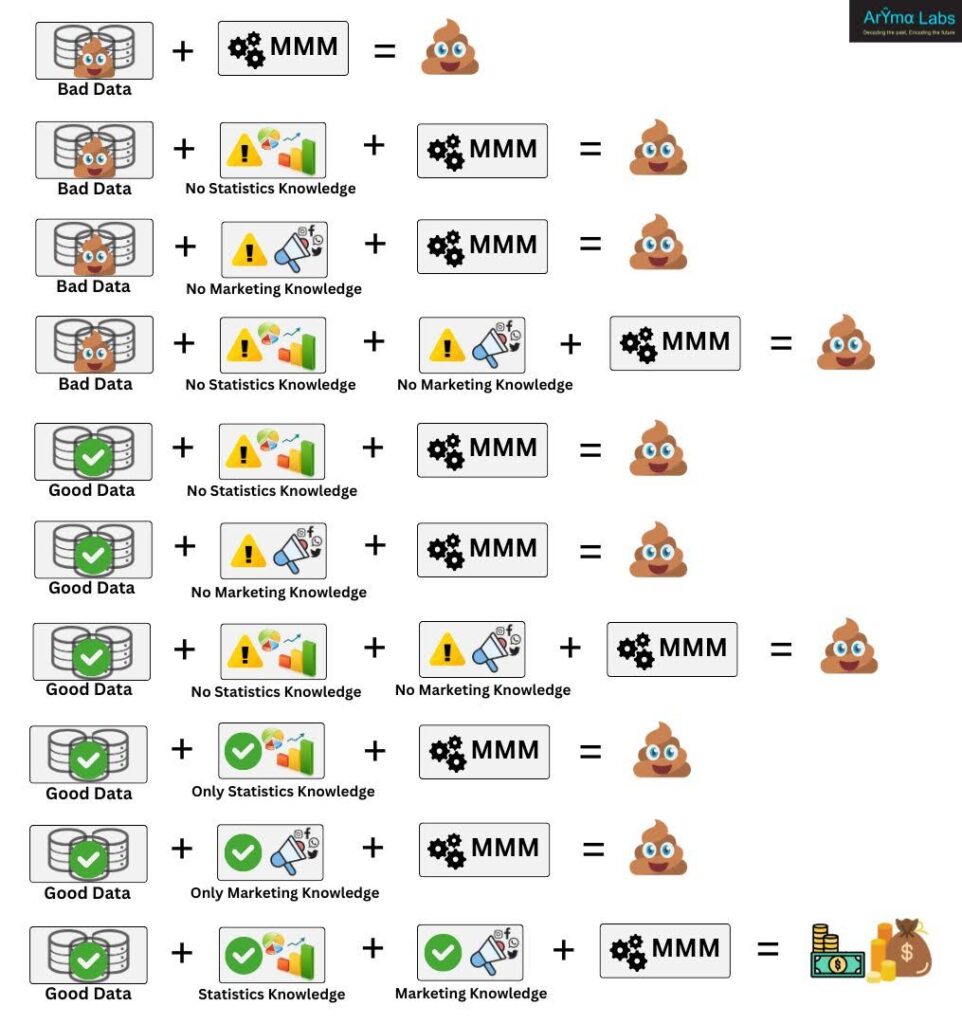
Data Collection and Management:
◦The ability to gather and organize large datasets from various marketing channels (online and offline), sales, pricing, and other relevant factors
◦Skill in ensuring data consistency and structure (time-series data is crucial for MMM)
◦Familiarity with data warehousing and ETL (Extract, Transform, Load) processes to prepare data for modeling (implied by the need for structured data).
Statistical Analysis and Econometrics:
◦A strong understanding of statistical concepts and econometric modeling techniques is fundamental to building and interpreting MMM models
◦Knowledge of regression analysis (used in traditional MMM)
◦Familiarity with Bayesian causal inference (used in platforms like Google’s Meridian)
◦The ability to evaluate the statistical significance of model outputs
Model Building and Customization (Especially for Open-Source MMM):
◦For platforms like Google’s Meridian and Meta’s Robyn, coding skills (e.g., in Python or R, the languages often used for statistical modeling and these open-source tools) are beneficial for customization, auditing, and refining models
◦The capacity to adjust model assumptions based on business knowledge and data insights
Data Interpretation and Visualization:
◦The skill to translate complex model outputs into clear, actionable insights for marketing and business stakeholders
◦Ability to create visualizations (charts, graphs) to communicate findings effectively.
Critical Thinking and Business Acumen:
◦The capacity to understand business objectives and marketing strategies to ensure the MMM model aligns with them
◦A skeptical mindset to question model results and identify potential biases or inaccuracies, as highlighted by Nico Neumann
. It’s important to recognize when results might be “too good to be true”.
◦The ability to connect model insights to budget allocation and optimization decisions
Knowledge of Marketing and Advertising:
◦A solid understanding of different marketing channels, their typical impact, and how they interact is essential for selecting relevant variables and interpreting their coefficients in the MMM model
◦Familiarity with marketing metrics such as reach, frequency, impressions, clicks, and conversions
◦Understanding of concepts like ROI and incrementality to integrate them into the MMM framework
Familiarity with MMM Platforms and Tools:
◦Experience with specific MMM software or platforms, whether they are traditional vendor solutions, open-source tools like Meridian or Robyn, or SaaS MMM platforms
◦The ability to input data, configure models, and extract reports from these platforms.
Outlook
The rise of MMM signals a potential shift away from relying solely on attribution methods. With the big digital platform players entering this territory the popularity of these tools will for sure flourish. Making this next step in an open source way is an exiting one and ensures a high degree in transparency and a low entry barrier to use the tools.
Leave a Reply